When Should You Not Invest in AI?Here are five situations where Artificial Intelligence is overrated.
ByGanes Kesari•
Opinions expressed by Entrepreneur contributors are their own.
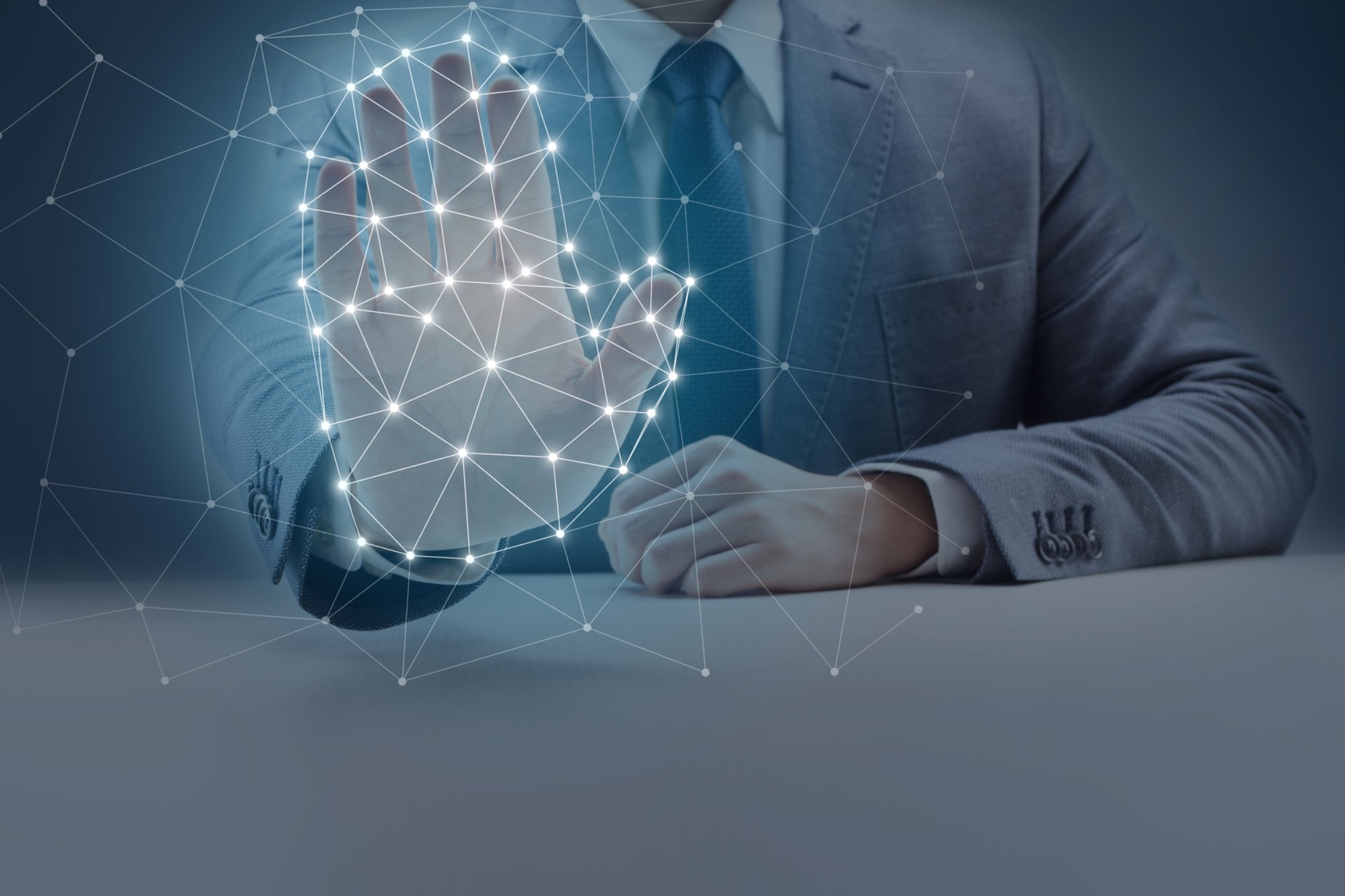
A study was conducted on the business adoption of Artificial Intelligence (AI) in the 1980s. Published in the MIS Quarterly, the study found that enterprises were rushing to invest in AI, and the projected market value was $4 billion.
然而,结果是令人震惊的。
The study found that over a five year period, just 33% of AI solutions delivered business value, while the rest were abandoned. Many popular applications of AI were proven to be pure hype and several companies became disillusioned with AI.
Today, the same story is repeating all over again.
Despite decades of progress in AI research and many recent breakthroughs, enterprises continue to struggle with adopting the technology. Asurveyby McKinsey found that only 8% of firms had practices that enabled them to adopt and scale AI.
AI has exceptional capabilities but it is not a fit for every situation. Here are five situations where it does not pay to invest in AI.
Related:What You're Buying Is Not Artificial Intelligence: How To Tell Fact From Fiction
1. When simpler solutions will get the job done
A majority of business problems can be solved by simple analysis. Even among organizations that use machine learning today, simple regression-based techniques are the most popular. Only a fraction of businesses really need AI. With AI capability getting democratized, it can be tempting to use it for every business problem. But, why use a cannon to swat a fly?
The $1 Million Netflix Prize was a global challenge to improve the accuracy of the Netflix movie recommendation engine by 10%. Netflixfound a winnerfrom over 50,000 global teams. They paid them the money, but never used their algorithm! Instead, they deployed a lower-ranked submission. Despite lower accuracy, this simpler solution had lower engineering costs and was more suitable for real-world use.
2. When you don't have enough data
Analytics techniques need data to discover actionable insights. The more powerful a technique is, the higher the volume of data it needs. AI has a huge data appetite and it needs hundreds of thousands of data points for basic tasks such as detecting pictures. This data must be cleaned and prepared in a specific format to teach AI. Unfortunately, a high volume of quality, labeled data is not a luxury that every organization can afford.
For example, AI can predict your sales for the next 4 weeks. But only if you have many months of granular, historical data. If all you have is the last few weeks' data, AI will not be able to help. So instead, use a simple forecasting technique like extrapolation. With just a few data points, it can give you credible insights to base your business decisions.
3. Where AI is still in experimentation
We are seeing spectacular advances in AI research every single day. Today, AI cangenerate picturesfrom your captions, or control a群无人机s. However, there is a big difference between doing stuff in carefully controlled scenarios, and performing tasks in the real world. Many of AI's impressive achievements are still in the experimental stages.
In 2013, the MD Anderson Cancer Center made a bold move todeploy IBM Watson'sAI to aid its clinicians in cancer diagnostics. After burning over $62 million over the next few years in trying to make it work in practical scenarios, the system wasfinally retired. Today, AI has gotteneven betterat detecting cancer but has yet to see mainstream adoption. While it is good to experiment with AI, you must know where it is ready for primetime.
Related:How AI Solutions Are Solving 5 Long-Standing Business Challenges
4. When the costs outweigh the benefits
今天,有人工智能领域可以做这项工作逢l. However, the total cost of ownership is so high that it may not be economically viable yet. When organizations think about AI, they often plan just for the implementation costs. These are just the proverbial tip of the iceberg. It takes a lot more investment to make AI work for you.
For example, if you're building an AI-driven customer experience platform, you must collect new data that captures deeper customer signals. You must manually label the data to train the AI, even before you spend on software platforms, high-grade hardware, andanalytics teams. Additionally, you must train end-users on data literacy, tailor your business workflows, and set aside a budget for ongoingchange management.
Toscale AI, you must budget as much for adoption as you spend on implementation. But, the big question is whether happier customers will earn you enough incremental revenue to cover all these costs of AI. Do this math upfront before you take the plunge.
5. Where you need understanding and empathy
Let's say your business problem doesn't fall into the above four scenarios. AI might still not be right for you if your users need understanding and care. AI is pretty good at pattern spotting and it can uncover deeper trends that humans have no chance of detecting. However, AIdoesn't understand a thing. Nor can it create an emotional connection with human users.
We saw how AI is breaking grounds in cancer research. It is beating diagnoses made by expert doctors. However, do you think patients are ready to hear about their cancer diagnosis from machines? Emotional intelligence and empathy are deeply human skills. How critical are these for your solution? Thinking about this question will help you decide whether you really need AI, and will show you the high level ofhuman involvementneeded, even if you deploy AI.
How not to put the cart before the horse
We've looked at the shortfalls of AI and situations where it can be a terrible fit. To place this in perspective, you must view AI as yet another tool in your analytics toolkit, albeit a powerful one.
How do you figure out if AI is the right fit for your needs?
Start with your business needs. Figure out ways in which technology and data can address the challenges. Frame the best solution to approach your business problem. Evaluate all the tools at your disposal, starting with the simplest techniques. As you move up in complexity, balance the simplicity and effectiveness of outcomes. If you pick AI, ensure that you have the right data, the needed budget, and the appropriate level of human intervention to make it work.
Related:4 Ways Artificial Intelligence Is Shaping the Future for Businesses Big And Small